Here is a brief summary of our recent paper from the University of British Columbia Environmental Physiology Laboratory authored by my colleague Dr. Assaf Yogev and our colleagues.
We think this is a paper that athletes & coaches can use to help understand uncertainty in performance data collection. The results here can be used as reference values which we can use to improve our confidence in how we are interpreting and prescribing our training.
This is a quick re-write of a twitter thread I posted when this study was first published in August, 2023. Since X née Twitter is more difficult to read these days without an account, and even less worth it than ever to create an account.. it might be worth transferring over some of my threads back here to the old blog. We’ll see!
Research Questions
We asked the question: how does the day-to-day variability of wearable near infrared spectroscopy (NIRS) muscle oxygen saturation (SmO₂) compare to other common physiological and performance markers used in cycling, including heart rate (HR), oxygen uptake (V̇O₂), blood lactate (BLa), and rating of perceived exertion (RPE)?
If we use NIRS in daily training or to monitor physiological change over time with training, how much day-to-day variation should we expect to see? How much of a change in observed values would we need to see to be confident that a ‘real change’ has occurred? i.e., how do we know if we have gotten fitter, or if it is just random fluctuation?
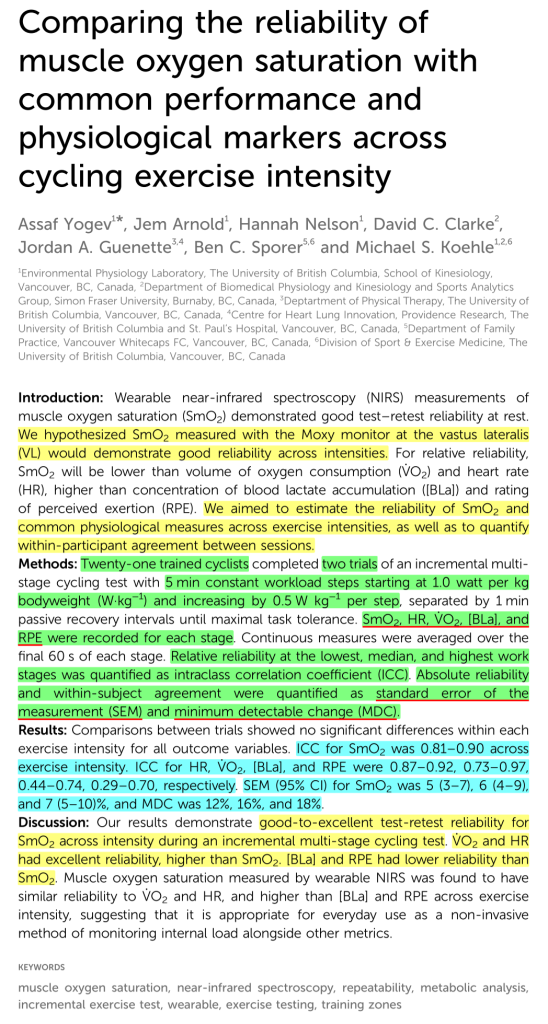
https://www.frontiersin.org/articles/10.3389/fspor.2023.1143393/full
We wanted to know how reliable was wearable NIRS compared to other common cycling metrics.
We looked at:
🔵Muscle O₂ saturation (SmO₂) at VL (quadricep) muscle
🔴Heart rate (HR)
🟣Systemic O₂ uptake (V̇O₂)
🟠Blood lactate (BLa)
🟢Rating of perceived exertion (RPE)
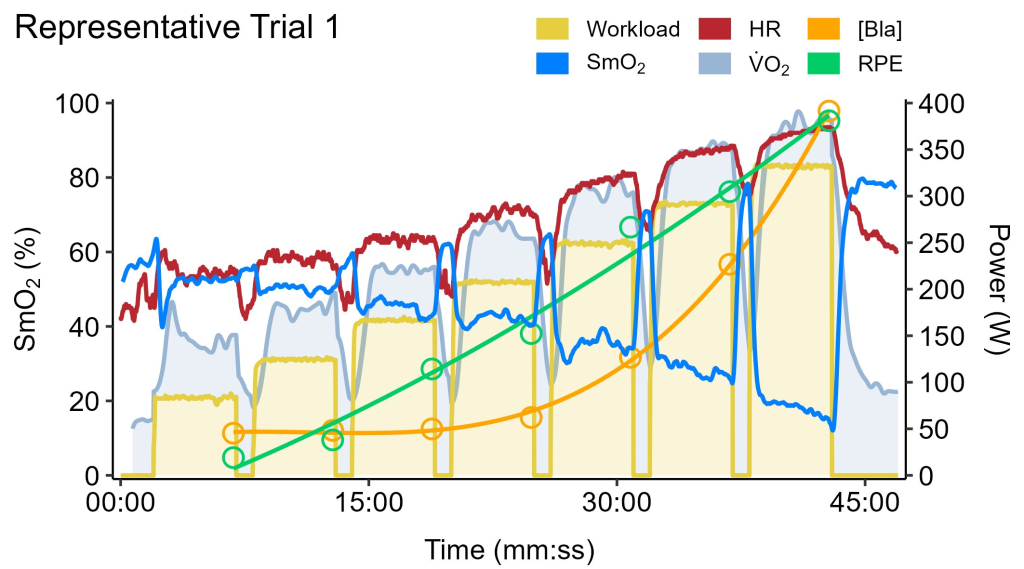
21 trained cyclists (10 F, 11 M) volunteered to participate in the study. Each athlete performed two identical assessments of a graded cycling test ‘5-1 protocol’. This included 5-minute constant workload stages starting at 1.0 W·kg⁻¹ and increasing by 0.5 W·kg⁻¹ per stage, to maximal task tolerance. 1 min rests between each work stage.
Trials were performed 1-2 weeks apart to replicate a typical repeated training session. Athletes were instructed to prepare for each training session as similarly as possible, like they would for an important training or test session.
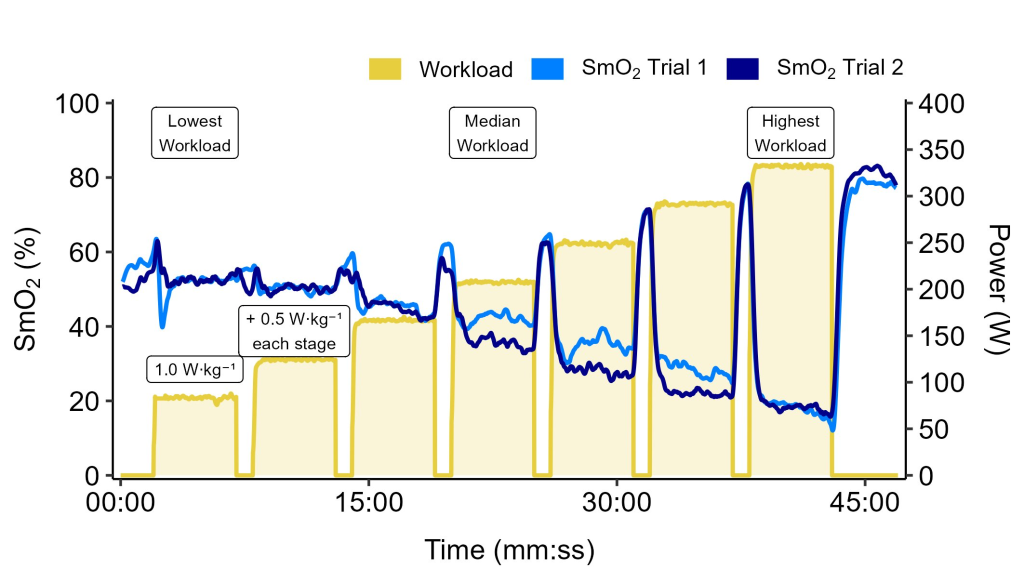
The athletes were of all different fitness and competitive levels, from amateur Cat 4 to national level cyclists. V̇O₂peak ranged from 46.8 to 73.9 ml·kg⁻¹·min⁻¹. This group had approximately 125% V̇O₂peak compared to population norms by sex, height, and weight (range 105 to 148% predicted V̇O₂peak).
Because of the very different fitness levels, athletes competed anywhere from 6 to 10 stages. We compared test-retest reliability and agreement at the first, median, and last stages, representing low, intermediate, and high intensity.
These intensities were not scaled to the athlete’s individual intensity domains, but should be broadly representative of responses across the intensity scale.
This representative athlete shows excellent SmO₂ agreement at low and high workloads, but higher variability at the median stage. This was common across most of the cycling metrics.
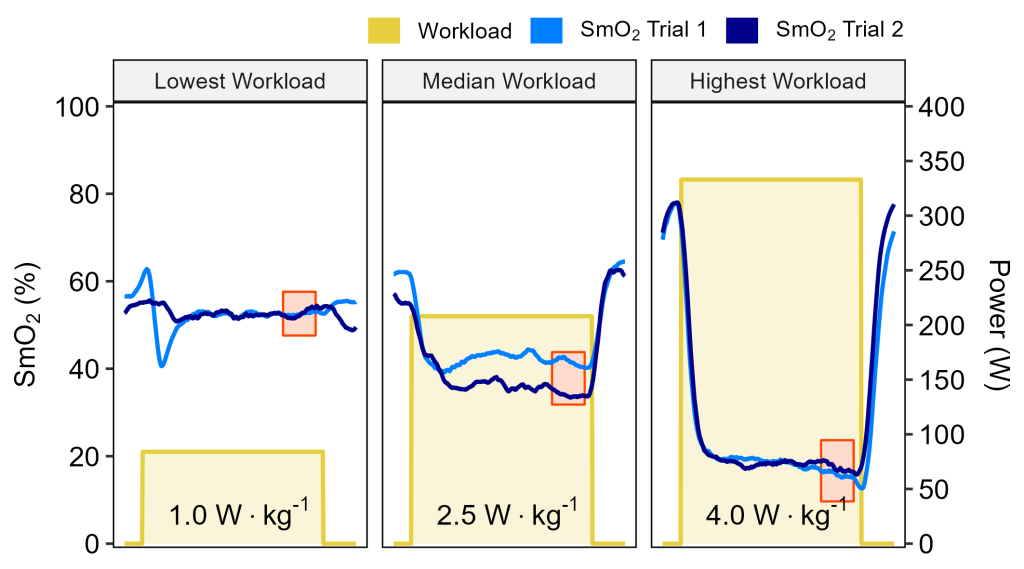
The Importance of Measurement Uncertainty
I think that we as athletes & coaches need to be aware of the uncertainty in anything that we are measuring and any data, qualitative or quantitative, that we rely on to make decisions.
Every observation has uncertainty – think of this as a range of values around the point estimate that we observe. This is often called a confidence interval (CI), because we can be very confident that the ‘real true value’ lays somewhere within this interval, but we cannot be confident where in that interval the value truly is. The real score might as well be any value within that range.
A larger range of uncertainty (wider CI) means we have lower confidence where the true score is. A smaller range of uncertainty (narrower CI) means we have higher confidence. I will get back to this concept…
Standard Error of the Measurement
One way to quantify the range of uncertainty for test-retest agreement, or how much we should expect a score to vary between any two sessions under similar conditions, is with the standard error of the measurement (SEM). SEM is like the standard deviation around a score that describes the range of values (in the units of the score itself) that we should expect if we take multiple repeated observations day to day or week to week, when we expect no real changes between observations.
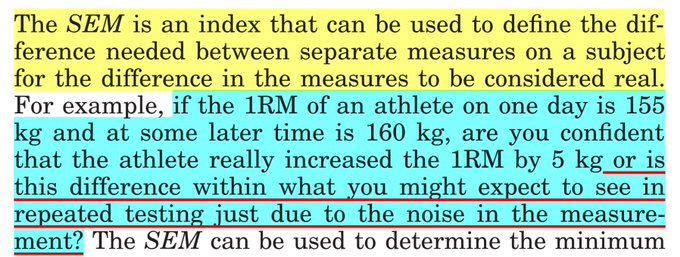
One of the go-to manuals on quantifying test-retest reliability & agreement
SEM were:
🔵NIRS ± 3-10 %SmO₂ at VL
🔴HR ± 2-5 bpm
🟠BLa ± 0.3-0.6 mmol·L⁻¹ at lower intensities, and ± 1.7 or greater at high intensity!
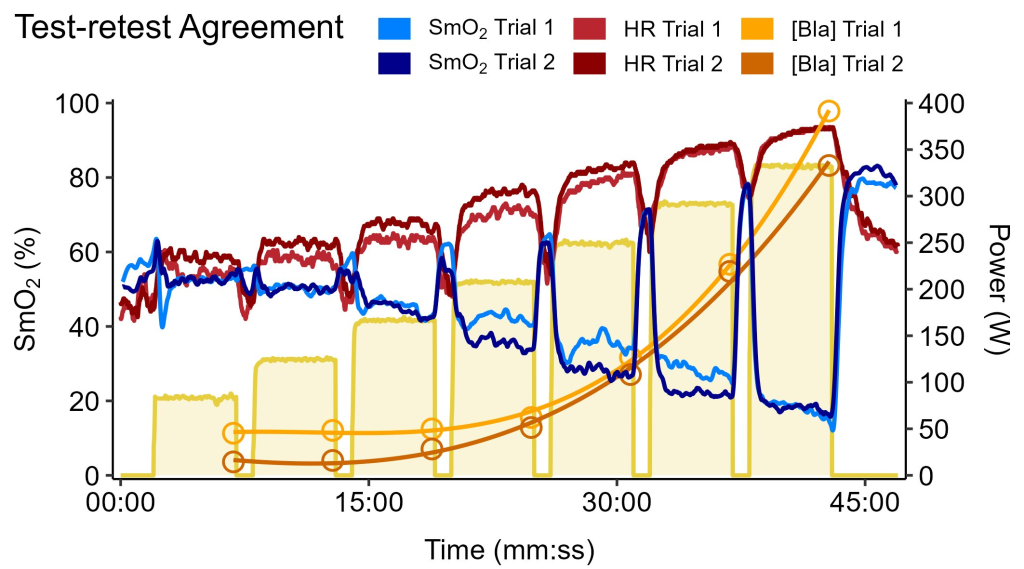
This suggests that, knowing nothing else about the athlete, we should expect scores from any given training session to the next to be higher or lower by around this amount.
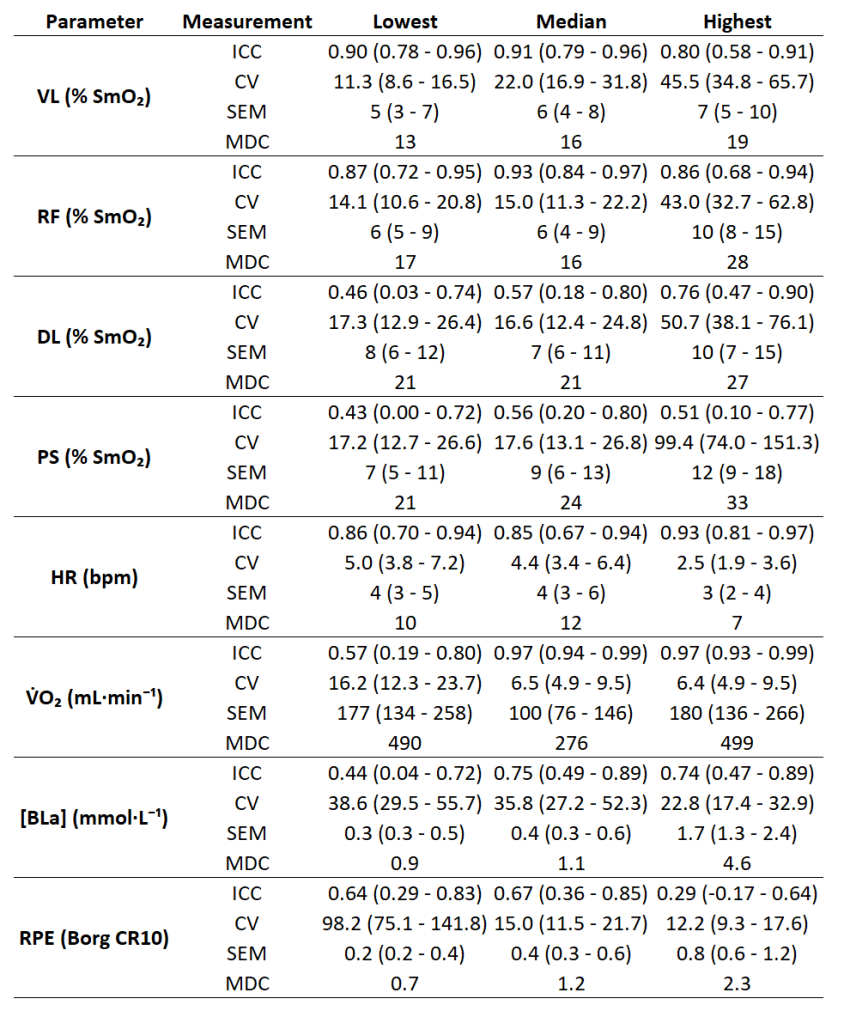
A difference within these ranges of uncertainty can be explained by random biological variation and measurement error. We can create stories to explain this variance – I slept poorly last night, I had a coffee before the ride, my favourite song was playing… – but we cannot attribute this change to a permanent shift in our physiology.
If I’m doing intervals at 300 W at a heart rate of 175 bpm one week, and the next week I’m doing the same workload at a HR of 170 bpm, I cannot be confident that my fitness has changed.
Not only is the difference of 5 bpm within the range of uncertainty (the SEM) for a single score, but remember each observation has their own range of uncertainty around them.
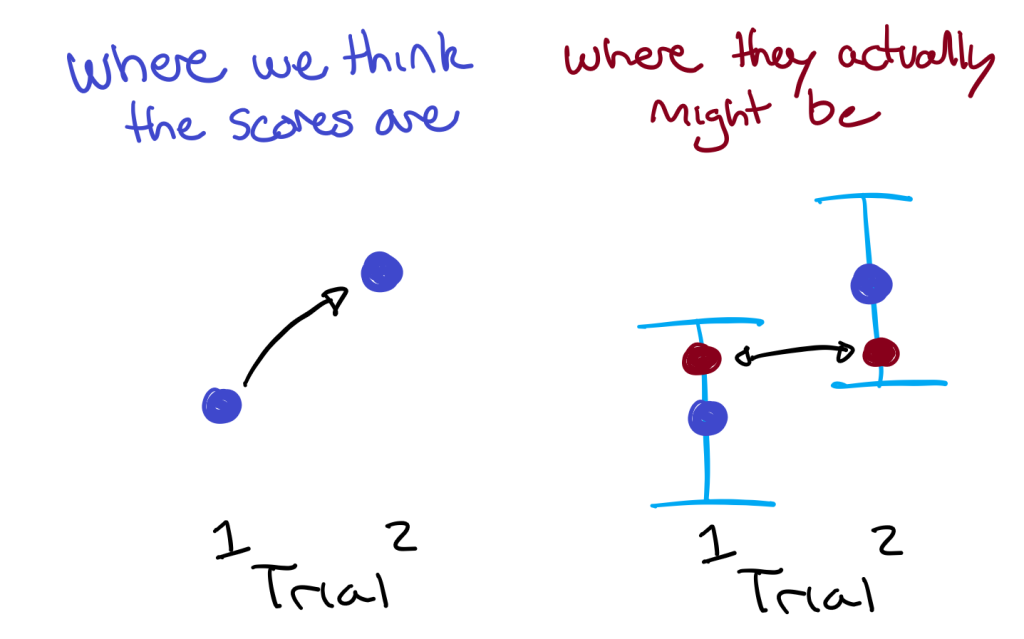
So how much of a change do I need to see to be confident that a ‘real’ change in my physiology/fitness has occurred?
Minimal Detectable Change
This is quantified by the minimum detectable change (MDC) (or other similar terms; minimal clinically importance difference, minimum detectable effect, etc.). MDC is like the 95% confidence interval around a score and describes by how much we would need to see a score change to be confident that a ‘real change’ has occurred.
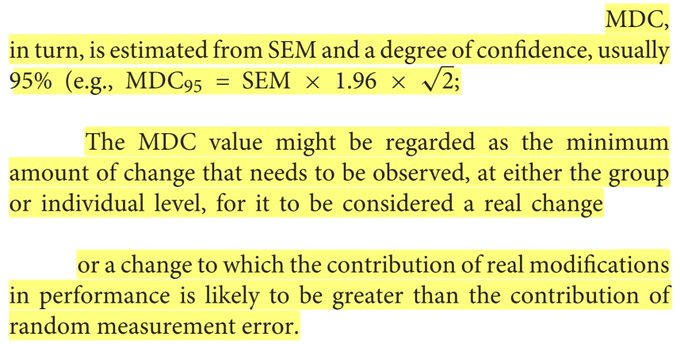
Think, if two scores each have a range of uncertainty, those ranges have to be non-overlapping in order to have confidence they are truly ‘different’.
MDC were:
🔵NIRS 13-19 %SmO₂ at VL
🔴HR 7-12 bpm
🟣V̇O₂ 275-500 ml·min
For HR & V̇O₂, these values are equivalent to around the average difference between any two sequential stages, i.e. 0.5 W·kg⁻¹. While for SmO₂ the difference is equivalent to ~2 stages, i.e. 1.0 W·kg⁻¹ difference.
This suggests that knowing nothing else about the athlete, if I repeated that 300 W interval after a training block to see if my fitness has improved, I would need to see a change from 175 to around 165 bpm to be confident my fitness had truly improved.
If I was testing V̇O₂max, I would want to see an increase by around 500 ml·min⁻¹ to be confident my VO₂max had improved.
Keep in mind, this is assuming we know nothing else about the athlete. These values are reasonable when we only have two observations: pre-test and post-test. Such as when we first start working with an athlete, or we first start using a new training gadget.
If we took repeated measurements, we would gradually improve our confidence in where the ‘real score’ is, and thus reduce our uncertainty to detect a ‘real change’ in that score.
This is the artistic science… or the scientific art? of coaching: making multiple repeated observations over time within a single athlete in order to improve our confidence and reduce uncertainty around any one observed value. This allows us to have more confidence in making decisions, prescribing training, predicting future observations, etc.
We can improve confidence in our data collection and interpretation a few different ways:
☝️controlling variables
✌️making more observations
🤟by knowing more context about our athletes beyond just the data!
I have higher confidence in face-to-face conversations than any other training or physiological data I might collect.
TLDR
- Every measurement has uncertainty.
- Uncertainty can give us information if we understand where it comes from, and how much we should expect.
- Data + context = information ⇒ application
Hopefully this paper gives qualitative and quantitative context for coaches, athletes, & sport scientists when interpreting NIRS and other common cycling metrics.
“Comparing the reliability of muscle oxygen saturation with common performance and physiological markers across cycling exercise intensity”
https://www.frontiersin.org/articles/10.3389/fspor.2023.1143393/full
Really interesting work. Can [Bla] be modeled from the SmO2%? Also, what do you think is causing SmO2% to recover to a higher % towards the end of the trial? I see SmO2 starts around 60% but peaks at around 80% despite dipping into the 20% range during the hardest effort? Do you think this is because of increased breathing? And congratulations on the publication.
LikeLike
Thanks!
Apologies for the brief answers for now. You’ll probably find I’ve written more helpful information about this topic elsewhere on this blog 🙂
Can [Bla] be modeled from the SmO2%?
Briefly, no.. because https://sparecycles.blog/2023/12/03/interpreting-group-level-data-for-individual-level-application/
Less briefly, but still very handwavy: it’s been attempted. SmO₂ suffers from being a very relative scale, while [BLa] is a bit more absolute(-ish)?… You know what, no they are both super relative 😅. SmO₂ values are more different between individuals than [BLa] values. Neither of them respond monotonically as a function of increasing exercise intensity, so we can’t even say that lower values in one are always associated higher values in the other. There is probably some complex over-fitted model that will predict one from the other in a homogenous dataset, but generally the answer right now is no, we cannot reasonably predict [BLa] from SmO₂ for the next marginal athlete that walks through my door to be tested.
what do you think is causing SmO2% to recover to a higher % towards the end of the trial?... Do you think this is because of increased breathing?
Well spotted. This is a commonly observed response. The short answer is that higher exercise intensity causes disruptions in the muscle metabolic milieu where we are measuring with NIRS; various substrate gradients are progressively disrupted (e.g. H+, K, PCr, pH, CO2, etc.). Those metabolites signal local vasodilation; expansion of local arterioles which increases blood flow (not what we are measuring with NIRS) and blood volume (kinda what we are measuring with NIRS) to the local tissue to try to compensate and meet the elevated energetic demand with greater O2 delivery. What we are seeing during recoveries (which are not discussed in this paper, but was very intentionally measured for hopefully future publications) is this ‘exercise hyperemia’, or reoxygenation kinetics during recovery intervals as a function of increasing exercise intensity.
This increasing trend in SmO2peak during recoveries isn’t always observed. Sometimes SmO2 will peak at lower values at higher intensities within the 1-min recovery windows. This is because reoxygenation kinetics are slowed after higher intensity; recovery takes longer / is less steep when the metabolic milieu is more disrupted. So if given enough time for complete SmO₂ hyperemia, we will most likely see higher SmO₂peak up to some plateau at high intensity, but the time it takes after each exercise interval to reach that peak will take longer.
Breathing is part of it, as of course ventilation is a pretty important integrated response in the system during exercise, which simultaneously responds to and contributes to the homeostasis of systemic (and therefore local) metabolic milieu. But there are probably more directly influential local mechanical and metabolic effects. NIRS is hyper-local looking at a small ~4 cm^3 tissue volume. So it will be related to the systemic response like everything is, but local things have larger effects on other local things usually before systemic effects need to be considered.
Great questions. Thanks!
LikeLike